
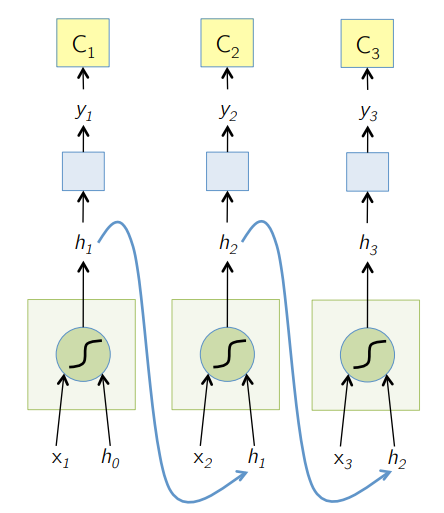
These MAR systems support user experiences by using MAR devices to provide universal accessibility to digital contents. MAR systems enable users to interact with MAR devices, such as smartphones and head-worn wearables, and performs seamless transitions from the physical world to a mixed world with digital entities. Mobile Augmented Reality (MAR) integrates computer-generated virtual objects with physical environments for mobile devices. We show that our method leads to improved lighting estimation while requiring less hyper-parameters compared to the state-of-the-art. Thereby, we propose a positional encoding that takes into account the camera calibration and ego-motion estimation to globally register the individual estimates when computing attention between visual words. For this task, we introduce a transformer architecture that is trained in an end-2-end fashion without any statistical post-processing as required by previous work. We tackle this problem by combining lighting estimates from several image views sampled in the angular and temporal domain of an image sequence. Recent work based on deep neural networks has shown promising results for single image lighting estimation, but suffers from robustness. Recovering the lighting is an inverse rendering problem and as that ill-posed. Photographs inherently encode information about the scene's lighting in the form of shading and shadows. In this work, we focus on outdoor lighting estimation by aggregating individual noisy estimates from images, exploiting the rich image information from wide-angle cameras and/or temporal image sequences. Extensive experiments demonstrate that our method can achieve superior performance to state of the arts. In this way, Soft-DA can effectively detect soft shadow with only a small number of soft shadow annotations. And then, we introduce a novel detector separation strategy to tackle the intention difference issue. Besides, to tackle obvious domain discrepancy and potential intention difference between different datasets and similar tasks, we first align data distributions between domains by feature adversarial adaptation. Note that we just only need to annotate 0.4K shadow masks for semi-supervised learning. Specifically, we create a limited soft shadow dataset, containing 1K soft shadow images with various scenes and shapes. Motivated by that soft shadow has similar characteristics with hard shadow, and many traditional hard shadow datasets are publicly available, we propose a novel soft shadow detection method (namely Soft-DA) based on adversarial learning and domain adaptation scheme. Existing shadow detection methods pay more attention to hard shadow scene since collecting and annotating hard shadow images is more effortless. Soft shadow is more challenging to detect than hard shadow due to its ambiguous boundary.
